When rapid iteration is the strategy
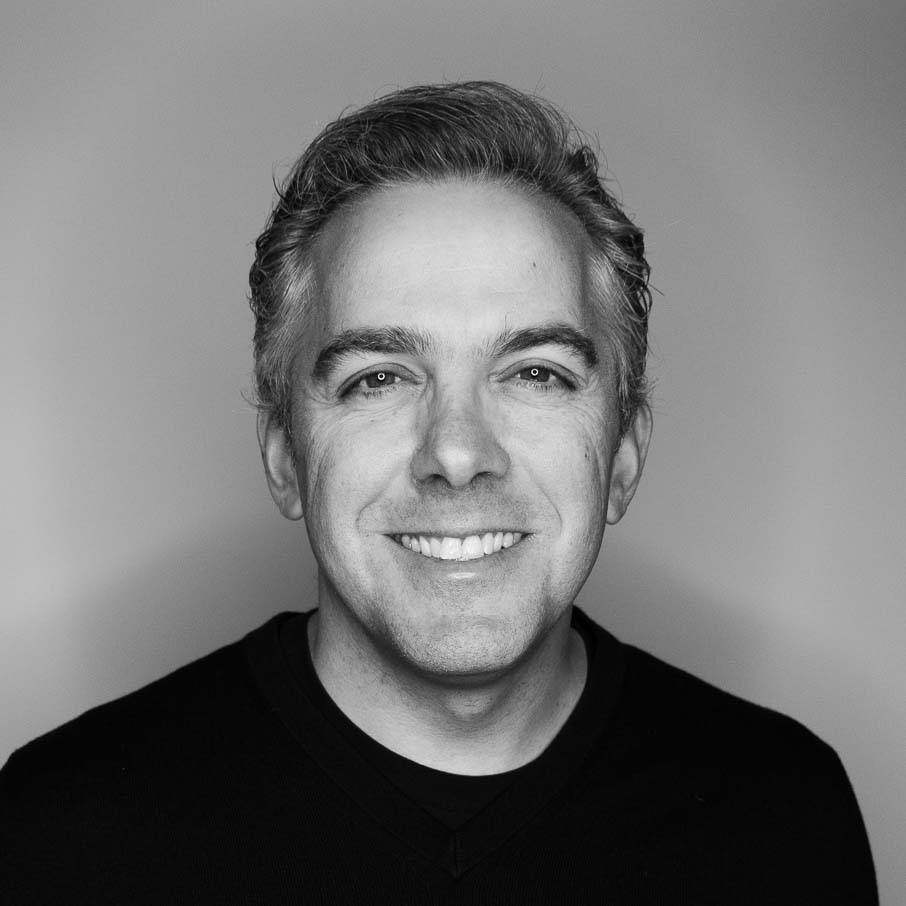
Elliott GrantFounder, CEO
| 5 min read
Michael Porter’s “What is Strategy?” argues that strategy is both choosing a coherent set of activities that are different to rivals’ and choosing what not to do when faced with tradeoffs. As a company born out of X, we seek out different perspectives, and search for new ways to approach big problems (see 10X is easier than 10%). But choosing what not to do can be difficult when dealing with highly uncertain, fast-changing technologies (such as machine learning). How can you know when to keep investing, and when to cut bait? How do you decide which path to take, when all are ‘less traveled’ and any could plausibly lead to a breakthrough?
What makes these decisions tricky is the ‘sunk cost fallacy’. In the sunk cost fallacy, future decisions are influenced by how much time and money has been already invested, despite the fact that that investment is gone. The Concorde is an archetypal example. The sunk cost fallacy is a result of commitment bias (keep doing what I’m doing), loss aversion (I don’t want to waste what I’ve done) and failure aversion (If I don’t follow through on a previous decision, it will be seen as a failure). These pitfalls have a sneaky habit of creeping in when working with fast-changing, unpredictable technologies. Because the future is unknowable, it is hard to prove that success might not be just around the corner!
While most companies use rapid iteration as a tactic to test out a theory or idea, at Mineral rapid iteration helps us choose what not to do (or what to stop doing). It isn’t just a tactic to move quickly — it is the strategy.”
The solution to this that we have embraced at Mineral is ‘rapid iteration.’ While most companies use rapid iteration as a tactic to test out a theory or idea, at Mineral rapid iteration helps us choose what not to do (or what to stop doing). It isn’t just a tactic to move quickly — it is the strategy.
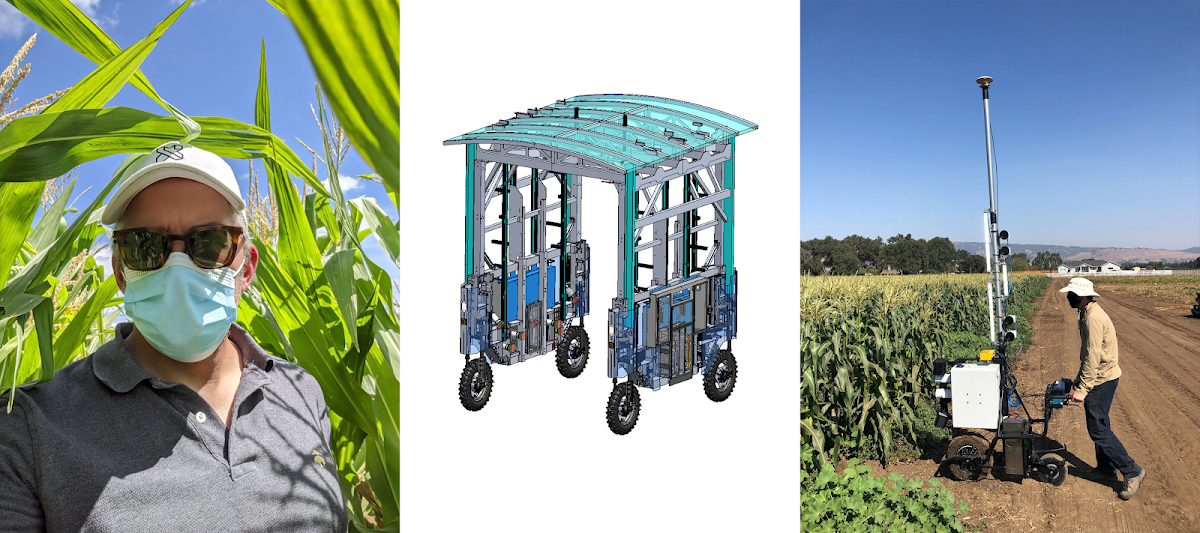
Problem: how to inspect 13’ tall corn? First Approach: convert our 5’ rover into a 13’ rover. Rapid Iteration Approach: quickly prototype a ‘skinny’ rover
Rapid iteration in practice
What does rapid iteration as strategy look like in practice? At Mineral we have created a culture of ‘done is better than perfect,’ of intentionally seeking real world feedback (and criticism) from experts in agriculture, and of accepting inefficiency as the price of flexibility. We invest in creating tools that help us go around the learning cycle faster — like developing a scheduling engine to manage all our computationally-intensive tasks. With that tool we can spend more time running experiments, and less time fiddling with AI job scheduling.
We hire people with curiosity and range — rather than narrow specialization. Specialists are more likely to fall into the sunk cost trap if they don’t also have range (“I earned my PhD in this topic… I want to apply it!”). We give data more weight than opinion in our decisions — something you’ll often hear in a Mineral meeting is, “Great idea! What’s the quickest experiment we can run to prove or disprove it?” We are also intentional about pursuing multiple paths in order to avoid becoming over-excited about one.
So how does rapid iteration solve the sunk cost fallacy? By going around a learning loop quickly, we invest as little as possible in each cycle — minimizing the sunk cost. For example, our experiments are designed to be cheap and scrappy: if we can learn something with a length of pipe, a mobile phone, a thrown-together app and some duct tape, then that’s what we’ll do first (like our ‘selfie-stick-phenotyper prototype’). We’ve found that it’s much easier to change direction if we haven’t over-committed (with time or money) to a particular idea. We’re also very clear with ourselves and our partners that the goal of these experiments is not to be right or wrong: the goal is to learn something. When we admit to ourselves that we don’t exactly know what the future looks like (and we’re probably wrong about it anyway), then it becomes easier to frame each iteration as learning, rather than a binary success/failure.
Quick experiments rarely give clear cut answers, but they sometimes give surprising ones … that lead to more experiments. For example, we decided to run an experiment using a CycleGAN to see if we could generate ‘deepfake’ images of strawberries. The resulting images wouldn’t have fooled anyone — but were nevertheless so remarkable that it launched a deeper investigation into using ML to synthesize plant images that could improve model performance in the real world.
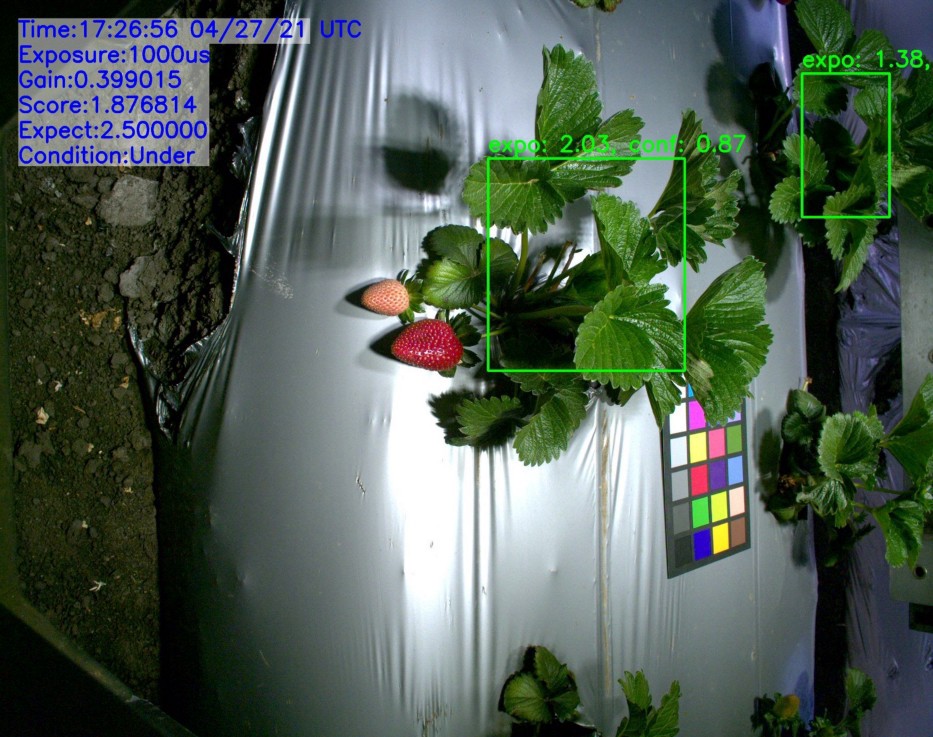
Our plant perception technology identifies strawberries using machine learning.
We think it makes sense to build an organization that is dynamically stable (like a bicycle) rather than statically stable (like a sofa).”
Spotting the sunk cost fallacy before it’s too late
At one point, we challenged our engineering team to design a version of our field rover that was twice as tall as the previous model so that we could capture images of corn, which can reach heights of 13 feet at maturity. The rover design we came up with was big, expensive and complicated. By anchoring on the idea that we needed to build a “tall rover” rather than a tool that would help us capture the imagery we needed, we were going slower round our iteration loop not faster — and running out of time before the corn season started. That’s when we realized we were in sunk cost territory. So we decided to scratch the super-sized rover idea altogether, and start over. We took our sensors and other bags of tricks off the rover, and hacked together something that could best be described as an electric wheelbarrow with an extendable 14 foot pole. Within weeks it was in the field, capturing data and teaching us about the core problems we wanted to solve — plant perception, not how to build a giant rover.
We’re not suggesting that a rapid iteration strategy is right for every company. In fact, quite the opposite. Carefully picking a single path and focusing on execution is a winning strategy for many companies — think running an airline, or scaling a chain of grocery stores. But in a domain like radical and long term innovation which has multiple layers of uncertainty, we think it makes sense to build an organization that is dynamically stable (like a bicycle) rather than statically stable (like a sofa).