Semantically robust unpaired image translation for data with unmatched semantics statistics
Zhiwei Jia, Bodi Yuan, Kangkang Wang, Hong Wu, David Clifford, Zhiqiang Yuan, Hao Su
| 5 min read
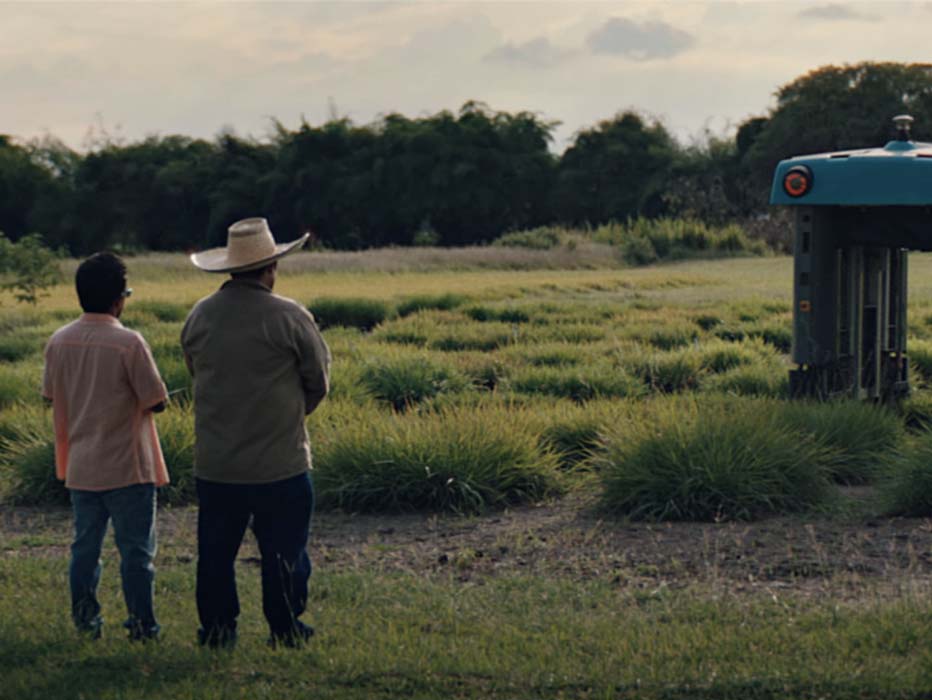
It takes a long time to label crop data. Imagine if we could expedite this step by using virtual crops.
Using machine learning (ML) to quickly analyze images of plants in the field has revolutionized phenotypic research: a process which used to take decades can now take days. But in order for ML models to work their magic, vast amounts of data must be meticulously labeled. What if there was a way to create a virtual simulation that matches the characteristics of plants in the real world? Think “deepfake” strawberries. These simulated plants can be used to train ML models, and they don’t need to be manually labeled by humans. In a simulated environment, researchers can experiment with different elements of perception, like lighting conditions, camera angles, plant diseases and more. It’s like shooting a movie on a soundstage as opposed to on location—the researchers control the variables.
This paper builds on the previous work of the team that developed the CycleGAN approach in 2017: a technique that involves the automatic training of image-to-image translation models without paired examples. CycleGAN allows practitioners to use a collection of images in order to create new images with specific stylistic differences. The team describes a new approach that improves the quality of the simulated images by encouraging perceptually similar image elements to be mapped to components with high semantic similarity, a property the authors call “semantic robustness.” This approach reduces the labeling effort required for training while maintaining high output quality, effectively closing the gap between simulated and real images.